Predictive coding networks for temporal prediction.
Our brains are constantly trying to predict the next moment based on current and past experiences. We developed a computer model that captures this ability of our brains to predict over time. Our model can learn and predict changes in the environment almost as accurately as advanced mathematical systems, but with a simpler approach that mimics natural brain activities. Importantly, when tested with natural movies, artificial "neurons" in our model behaved similarly to actual brain cells that detect motion.
One of the key problems the brain faces is inferring the state of the world from a sequence of dynamically changing stimuli, and it is not yet clear how the sensory system achieves this task. A well-established computational framework for describing perceptual processes in the brain is provided by the theory of predictive coding. Although the original proposals of predictive coding have discussed temporal prediction, later work developing this theory mostly focused on static stimuli, and key questions on neural implementation and computational properties of temporal predictive coding networks remain open. Here, we address these questions and present a formulation of the temporal predictive coding model that can be naturally implemented in recurrent networks, in which activity dynamics rely only on local inputs to the neurons, and learning only utilises local Hebbian plasticity. Additionally, we show that temporal predictive coding networks can approximate the performance of the Kalman filter in predicting behaviour of linear systems, and behave as a variant of a Kalman filter which does not track its own subjective posterior variance. Importantly, temporal predictive coding networks can achieve similar accuracy as the Kalman filter without performing complex mathematical operations, but just employing simple computations that can be implemented by biological networks. Moreover, when trained with natural dynamic inputs, we found that temporal predictive coding can produce Gabor-like, motion-sensitive receptive fields resembling those observed in real neurons in visual areas. In addition, we demonstrate how the model can be effectively generalized to nonlinear systems. Overall, models presented in this paper show how biologically plausible circuits can predict future stimuli and may guide research on understanding specific neural circuits in brain areas involved in temporal prediction.
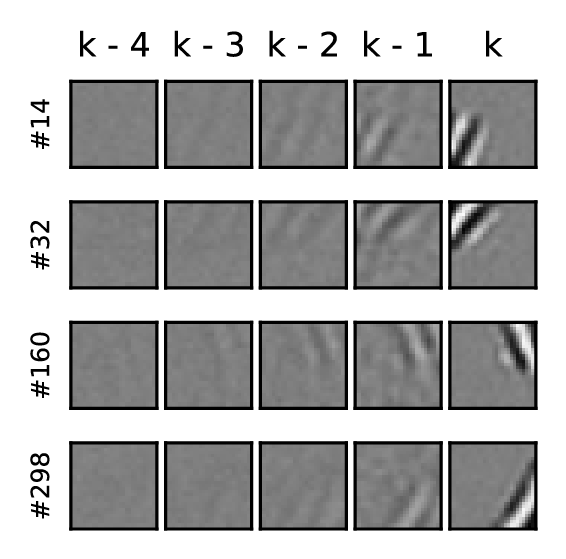
2024. PLoS Comput Biol, 20(4)e1011183.
2023. Adv Neural Inf Process Syst, 36:44341-44355.
2021. Cogn Affect Behav Neurosci, 21(6):1196-1206.
2023. PLoS Comput Biol, 19(4)e1010719.